Ambassador post originally published on the InfraCloud blog by Atulpriya Sharma, CNCF Ambassador, Sr. Developer Advocate, InfraCloud Technologies
Machine Learning (ML) has revolutionized various industries by enabling data-driven decision-making along with the automation of certain tasks. For instance, many banking institutions deploy advanced machine-learning models to detect fraudulent transactions. These models need to evolve constantly otherwise there will be a steep rise in false positives.
However, deploying new machine learning models in production can be challenging. Training the model on production data, deploying it, and maintaining it isn’t easy. Many a time, machine learning models in production fail to adapt to the changing data and environment. And doing all of it manually isn’t efficient.
MLOps or Machine Learning Operations helps solve such challenges by automating the complete lifecycle of machine learning development and deployment. In this post, we will talk about MLOps, how it works, its benefits and challenges, and various tools that you can use.
But before we dig into what MLOps is, let us take a step back and look at the challenges with the existing model or working with machine learning models.
Machine Learning Development: Existing Challenges
Though we have been advancing in the Artificial Intelligence (AI) and machine learning space, the evolution is slow-paced when it comes to processes associated with it. Many organizations today deploy machine learning models for various use cases, however, most of these processes are manual that bring in multiple challenges.
- Data Quality and Management: Incomplete or incorrect data can affect the performance of a machine learning model. Manually working with multiple data sources and formats is error-prone and slows down the process.
- Model Complexity: With changing data and environments, machine learning models can become complex over time. Deploying, scaling, and working with complex models is challenging.
- Reproducibility: Due to the variation in data, environments, infrastructure, etc., it’s difficult to manually reproduce machine learning models and track changes over time.
- Deployment Complexity: Deploying machine learning models on different environments and systems can be complex and may require significant changes in your infrastructure.
- Collaboration: Machine learning development teams usually have people with different expertise – data scientists, developers, and operations staff. And without a streamlined process, these three would work in silos creating their own set of processes, leading to misunderstandings.
These are a few challenges that hamper the development and deployment of machine learning models. To overcome these challenges, MLOps was introduced which automates and streamlines various processes.
What is Machine Learning?
Machine learning as we all know is a branch of Artificial Intelligence that enables computers to learn & make predictions without actually programming them.
Machine learning is used across different industries. From providing you with a recommendation of a movie to watch (YouTube/Netflix) to understanding your voice and turning on a fan (Alexa), machine learning is used widely in our daily lives. Even industry sectors like banking use it to detect fraudulent activity on credit cards and deploy chatbots to make customer service faster. These are just a handful of applications of machine learning in real life.
Types of Machine Learning
Just like we humans learn in different ways, there are means by which algorithms learn as well. Below are the three main forms of machine learning. There are three types of machine learning:
- Supervised: Supervised learning is the type of machine learning where the input data provided is labeled. This means that some data is already tagged with the correct answer. For example, if you’re providing input with images of fruits, you’ll also label each image with the corresponding fruit. The model will learn from this and be able to produce correct output for new data based on the labeled data. Regression and classification are two types of supervised learning models.
- Unsupervised: Unsupervised learning is where the input data provided is neither labeled nor classified. The algorithm has to act on this without any information. Here, the model has to group unsorted input according to patterns, and similarities. For example, you can provide input with images of fruits that the algorithm has never encountered without any labels. The algorithm will group/categorize the fruits based on the patterns it identifies. Clustering and Association are two types of unsupervised learning models.
- Reinforcement: Reinforcement learning is where the algorithm learns based on feedback. It learns to behave in an environment & gets feedback based on its actions. It performs actions using this trial-and-error method and learns over time.
Machine Learning Models
At the heart of machine learning are machine learning models. Algorithms that are trained on datasets and optimized to make accurate predictions on new data. The output of this process is a machine-learning model.
Based on the way these models predict, there are different types of machine learning models:
- Regression Models: These models are used to predict numerical values. They find relationships between dependent and independent variables to arrive at a prediction. For example, predicting the stock price based on historical data is a regression model. Linear regression is one of the most widely used regression models.
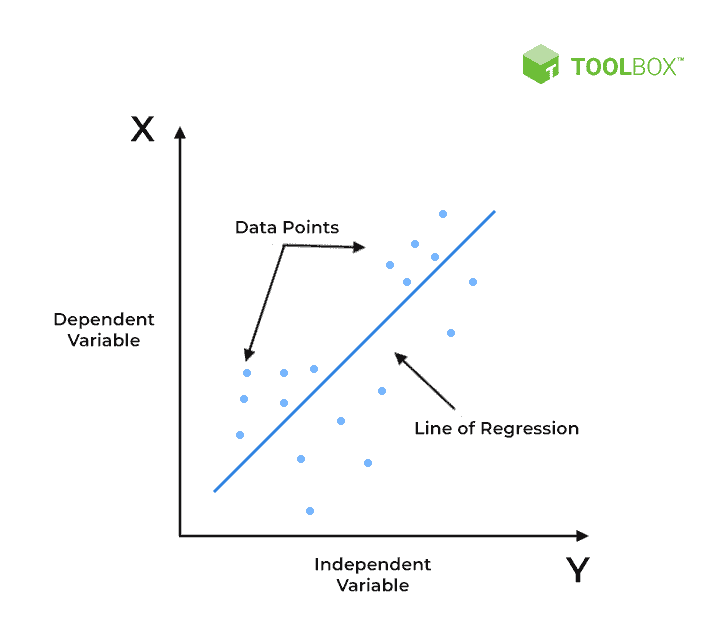
- Classification Models: These models help predict a category value. These find patterns in data and help categorize them into specific categories. For instance, classifying emails as spam or not is done by a classification model. Decision trees and random forests are popular classification models.
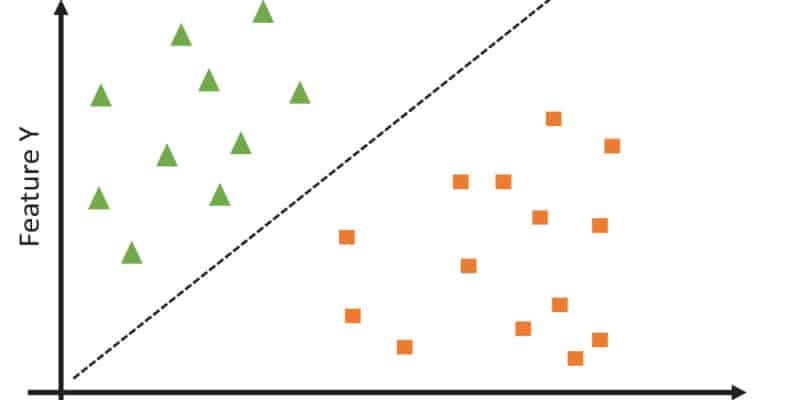
- Clustering Models: These models group data points together based on their similarities. These work by identifying patterns and grouping similar data points into clusters. K-Means is a popular clustering model. For example, grouping customers based on their purchase history is done using clustering models.
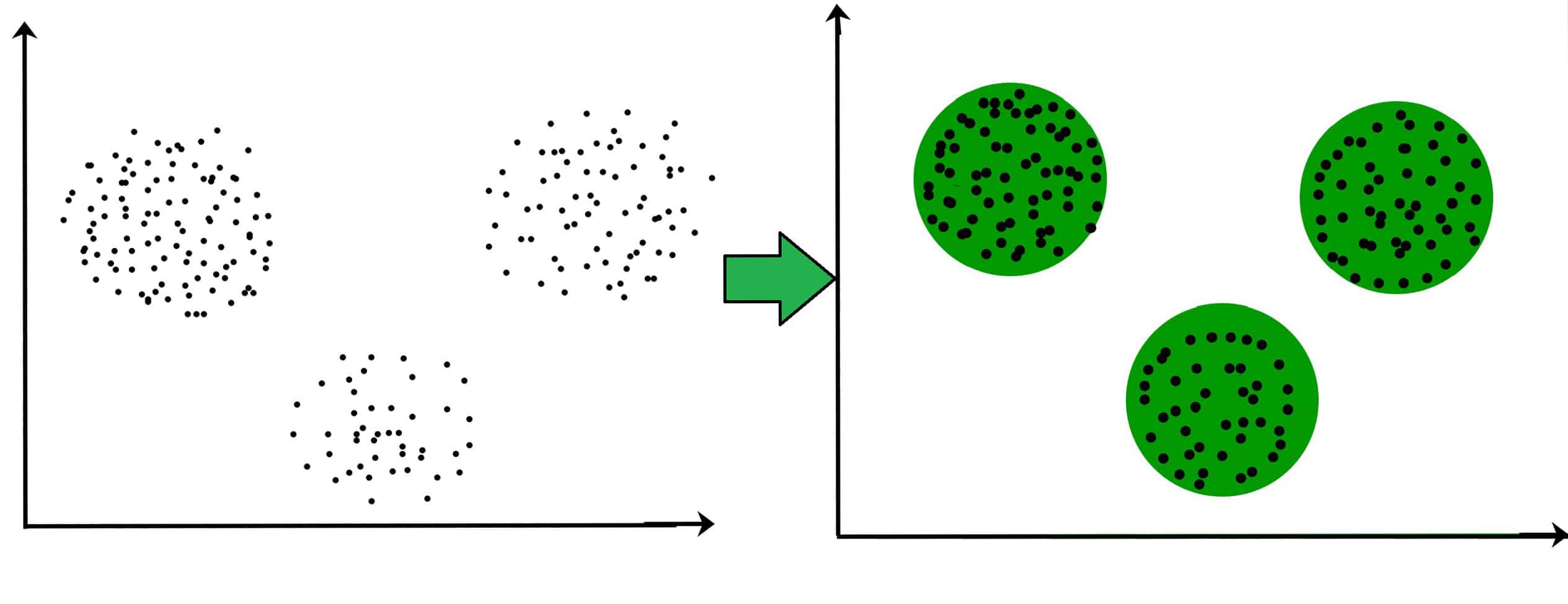
- Neural Network Models: Inspired by the human brain, these models are used for complex tasks that include speech and vision. They work by using layers of artificial neurons to identify complex patterns in data. Convolutional Neural Network is one of the most popular neural network models and is used widely for image recognition.
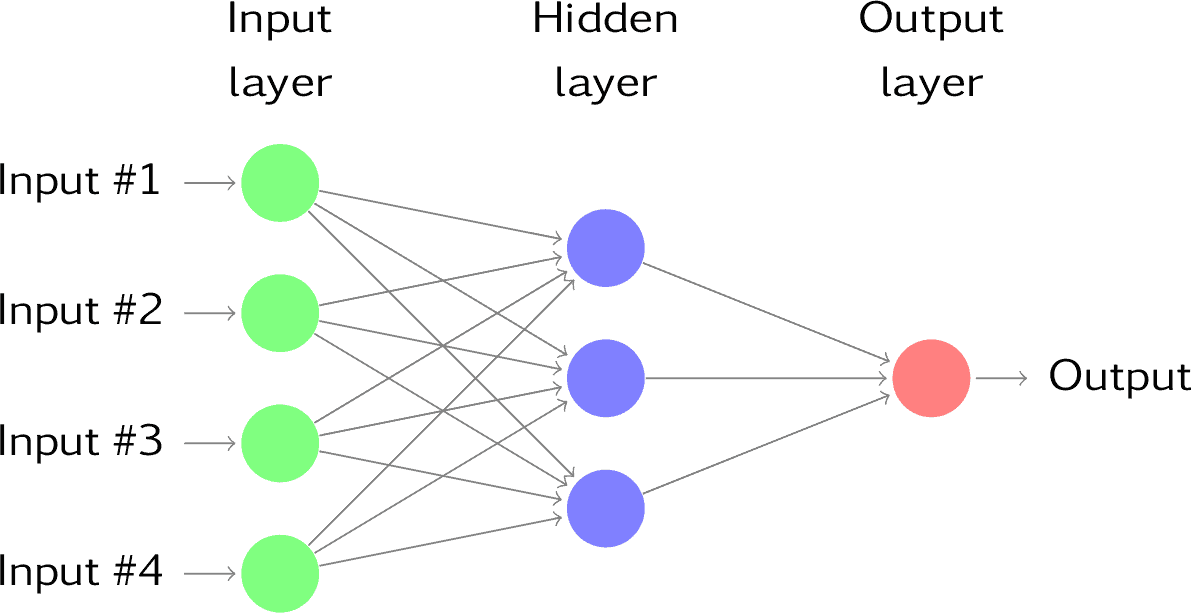
What is MLOps?
MLOps or Machine Learning Operations provides a framework for creating a consistent and reproducible machine learning pipeline. It helps streamline the process of developing, deploying, and managing machine learning models. MLOps is the practice of applying DevOps principles and practices to machine learning workflows.
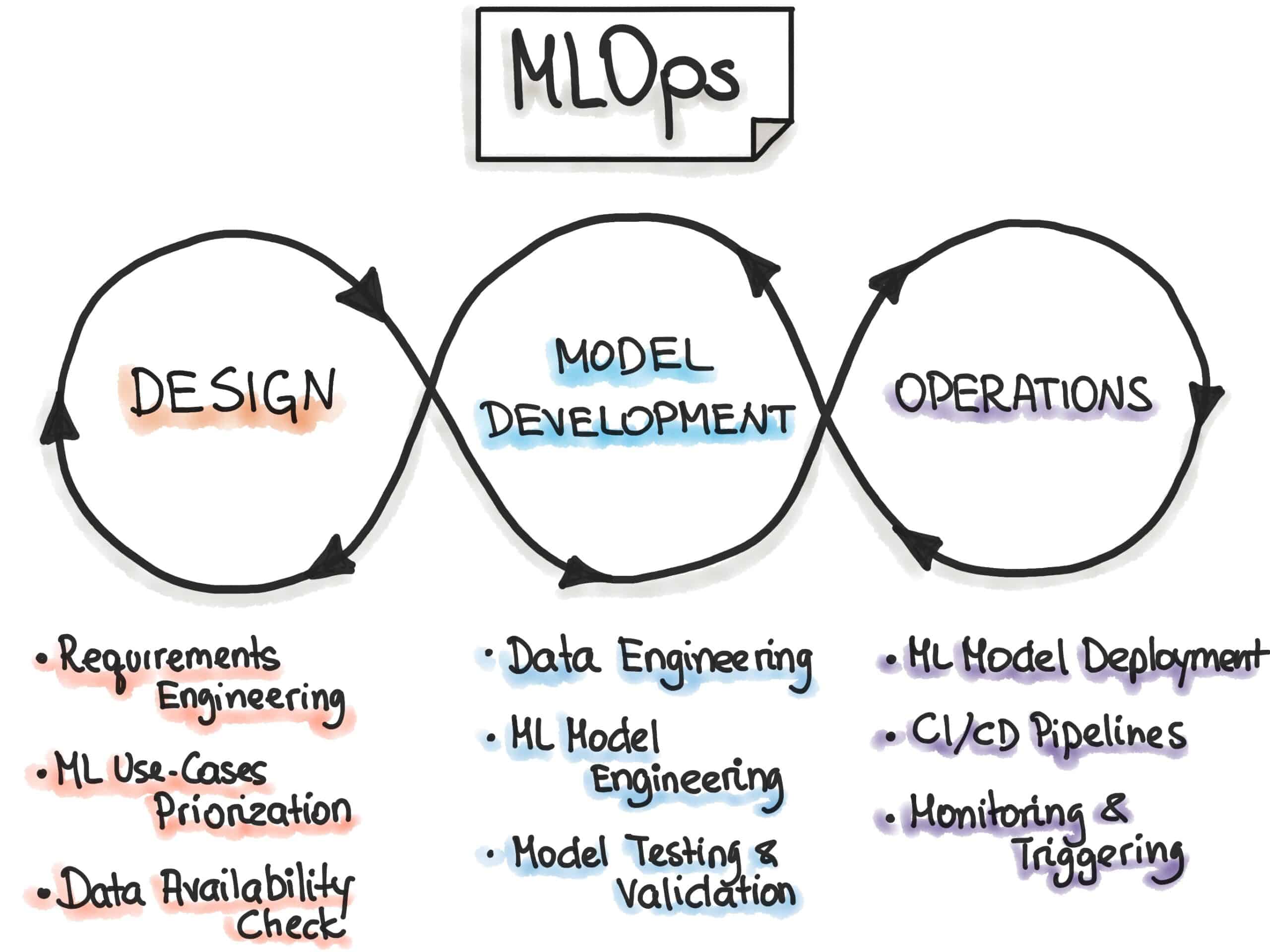
With the help of MLOps, data scientists, engineers, and operations teams can collaborate more effectively – leading to better workflow management, increased productivity, and faster time to market. It’s the way forward and in fact, a report by IDC says that by 2024, 60% of enterprises will have their MLOps processes operationalized using MLOps capabilities.
MLOps Workflow
MLOps pipelines refer to the end-to-end processes and tools used to manage, deploy, monitor, and maintain machine learning models in production environments. A robust MLOps pipeline ensures that machine learning models can be updated, scaled, and maintained efficiently, while also meeting business requirements for accuracy, reliability, and speed.
MLOps pipelines typically include steps such as data ingestion, data preprocessing, model training, model testing, model deployment, and model monitoring.
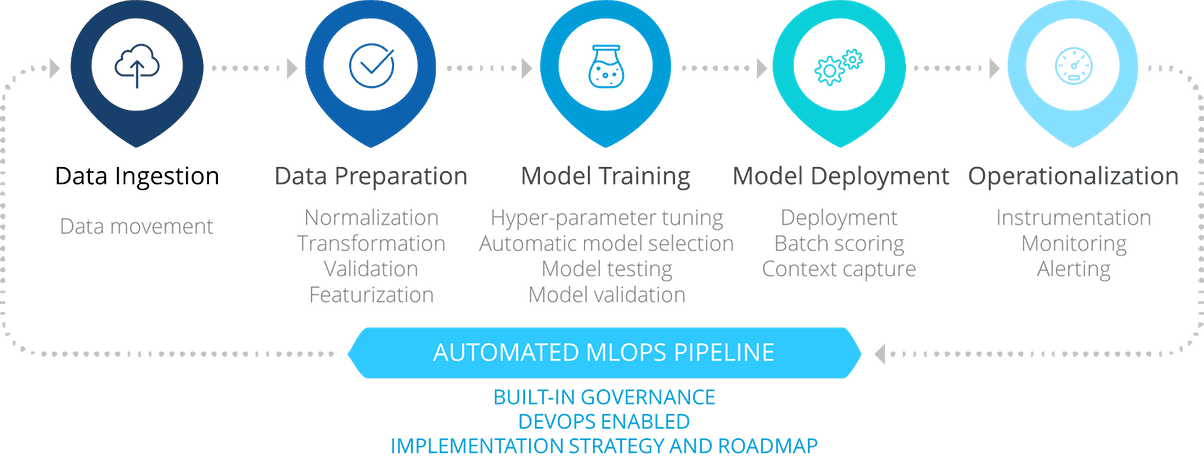
#1 Data preparation and management
The first phase in any machine learning process is the collection of data. Without clean and accurate data, the models are useless. Hence, data preparation & management is a crucial phase in MLOps workflow.
It involves collecting, cleaning, transforming, and managing data so that we have the correct data in place to train the ML models. The end goal is to have data that is complete and accurate.
Let us deep dive into the finer details of the sub-processes involved in the data preparation and management phase:
- Data Collection: This step involves the collection of data from multiple sources. The incoming data can be structured, unstructured, or semi-structured. It could come in from APIs, databases, or any other sources.
- Data Cleaning: Once the data is collected, the next step is to clean it. There can be missing values, duplicates, outliers, etc. in the incoming data. The objective of this process is to ensure that only high-quality data is fed to the models for better accuracy.
- Data Transformation: The clean data is then transformed or integrated. Processes like normalization, aggregation, feature extraction, etc. are applied to make it suitable for the machine learning model.
- Data Versioning: Since data is evolving, it’s important to keep a track of it. That’s what happens in the data versioning phase. Data is versioned here so that it is traceable and the machine learning model becomes reproducible.
- Data Visualization: Data visualization involves generating meaningful visualizations from the data. This helps to identify patterns and insights that can help make the machine-learning model better. It also helps to communicate the outputs of a model to various stakeholders.
- Data Governance: The process of data governance helps ensure that the data is compliant with various guidelines and laws like GDPR and HIPAA. It also ensures that data is handled in the correct manner following the best practices and security guidelines.
#2 Model training and validation
Once you have the data ready, you can feed it to your machine-learning models to train them. This is where you ensure that your models are trained well, and validated to ensure they perform accurately in production.
Steps involved in model training and validation include
- Data Splitting: Before training any machine learning model, it’s important to split the data into three subsets: training, validation, and test data. The training data will be used to train the model, validation data is used to test the performance of the model and the test data will be used to validate the performance of the model.
- Model Selection: This step involves choosing the right machine learning algorithm that can solve the problem at hand. The selection of the algorithm is done on the basis of the problem, type, and amount of data along with the desired accuracy.
- Model Training: This step involves training the chosen model with the training data. Various parameters involved are modified to get an accurate result.
- Model Validation: Once the model is trained, it’s time to validate it. Using the validation data set, the model is validated for its performance and accuracy. Various metrics like accuracy, F1 score, confusion matrix, etc. are used to determine the performance of the model.
- Model Optimization: This step involves optimizing the performance of the model by adjusting its hyperparameters. Hyperparameters are variables whose values are set before training the model. This is used to control the learning behavior of the model.
#3 Model deployment
After the model is trained and the performance is validated, it’s time to deploy it to production. The model deployment phase involves taking the model that has been trained, tested, and validated in earlier phases and making it available for use by other applications or systems.
The process of deployment involves several steps as follows:
- Model Packaging: The first step is to convert the model into a format that other applications and systems can use. In this phase, a model is packaged with a serialized format either as a pickle or JSON along with the dependencies.
- Containerization: The packaged model is added to a container along with the dependencies. Containerized models are easy to deploy and manage across multiple environments and systems.
- Deployment to Production: The containerized model is then deployed to the production system. This can be on a cloud-based platform like AWS, Azure, or GCP, or in an on-premise setup.
- Model Scaling: Once the model is deployed to production, it may be necessary to scale the model to handle large volumes of data and increase usage. This may involve deploying the model to additional nodes and using a load balancer.
#4 Continuous model monitoring & retraining
Machine learning models aren’t something like deploy and forget. One needs to constantly monitor the model’s performance and accuracy. Since the real world can change which might affect the efficiency and accuracy of the model.
Various sub-processes involved in model monitoring are
- Data Collection: The first step involves the collection of real-world data from production. This could be system metrics, user interactions, or anything that will be useful to evaluate the performance of the model.
- Model Performance Evaluation: The production data is then used to evaluate the performance of the machine learning model. Once again, metrics like accuracy, F1 score, confusion matrix, etc. are used to evaluate the performance.
- Anomaly Detection: Based on the results obtained in the above step, one needs to detect if there are any anomalies. Results from the previously used historical data can be compared with the current one and the deviation can be noted.
- Model Update: Once an anomaly has been detected, the next step is to update the machine learning model. This would involve all the steps discussed in the model training and validation phase.
There are tools available for each of the processes mentioned above. You have the option to choose from proprietary tools to open source ones. To make your lives easier, here’s an Awesome MLOps repository that has a list of open source MLOps tools categorized for each step.
We saw the various processes involved in a typical MLOps workflow. Each of these stages helps streamline the process of model deployment. Adopting MLOps can be challenging, but the benefits are substantial in unlocking the potential of machine learning for driving innovation and business outcomes.
Benefits of MLOps
By now, we know that MLOps helps streamline the machine learning development process. However, it also comes with a suite of benefits.
Some advantages of implementing MLOps are:
- Improved Efficiency: MLOps removes unnecessary manual steps and automated repetitive tasks making the whole process more efficient and reliable. This helps reduce development time and costs.
- Version Control: MLOps provide version controls for machine learning models and data. This helps organizations track the changes and even reproduce the model when required.
- Automated Deployment: Organizations can deploy machine learning models faster by implementing MLOps and bringing down the deployment times.
- Enhanced Security: The entire MLOps process can be safeguarded with access controls, data, and model encryption techniques for added security.
- Improved Collaboration: It enables seamless communication with different teams thus leading to improved collaboration.
- Faster Time to Value: With MLOps, organizations can deploy their machine learning projects faster – leading to faster time to value for their customers.
Summary
In the age when generative AI is booming, the future of machine learning is bright. It has immense potential to deliver enormous business value. However, it cannot happen just by building newer machine learning models. You need a comprehensive end-to-end process that takes care of the entire machine learning lifecycle from data preparation to model deployment and monitoring. By adopting MLOps, organizations can deliver value faster, optimize costs as well as improve efficiency.
By now you would have some understanding of what MLOps is and its benefits. Keep an eye on our upcoming blog posts where we’ll talk about MLOps on Kubernetes and associated tools.
That’s about it for this blog post, feel free to reach out to Atul for any suggestions or queries regarding this blog post.
References: